Causal trees bootstrapping and max_leaf_nodes
fixes with minor update
#583
Add this suggestion to a batch that can be applied as a single commit.
This suggestion is invalid because no changes were made to the code.
Suggestions cannot be applied while the pull request is closed.
Suggestions cannot be applied while viewing a subset of changes.
Only one suggestion per line can be applied in a batch.
Add this suggestion to a batch that can be applied as a single commit.
Applying suggestions on deleted lines is not supported.
You must change the existing code in this line in order to create a valid suggestion.
Outdated suggestions cannot be applied.
This suggestion has been applied or marked resolved.
Suggestions cannot be applied from pending reviews.
Suggestions cannot be applied on multi-line comments.
Suggestions cannot be applied while the pull request is queued to merge.
Suggestion cannot be applied right now. Please check back later.
Proposed changes
Hi, my fix is aimed to solve recent causal trees issues.
I also added several updates to make causal trees more transparent and flexible for various tasks.
CausalRandomForestRegressor
model had incorrect boostraping because of the wrong samples weightning mechanism.Code details are here.
In general, this happened because samples weights are not used in causal trees as they are in common sklearn tree regressors.
Now instead of using
np.bincount(indices, minlength=n_samples)
as weights multiplier_parallel_build_trees
function generates the views of bootstraped X, y, treatment arrays. See here.Non-default values of
max_leaf_nodes
don't break tree fit. To fix the issue CausalTree regression with 'max_leaf_nodes=xxx' doesn't work #567 sklearnBestFirstTreeBuilder
class was modified into internalBestFirstCausalTreeBuilder
.min_samples_split
parameter got updated behaviour based on treatment and control sample sizes in each tree node. Details can be found in DepthFirstCausalTreeBuilder and BestFirstCausalTreeBuilder. The fix is aimed to solve the issue of infinity node values which may happen in case of high imbalance of treatment and control sample size.sklearn version in
setup_requires
setup.py
was updated to<=1.0.2
from>=0.22.0
. Probably, the related issue is binary incompatibility. Expected 352 from C header, got 328 from PyObject #581.Minor update:
DepthFirstCausalTreeBuilder
andBestFirstCausalTreeBuilder
were improved to recognize a node split as a leaf with the use of auxiliary information about the number of treatment and control samples. This may be crucial in some bootstrapping cases during CausalRandomForestRegressor fit.Please, see here and here.
New
groups_penalty
parameter inCausalTreeRegressor
andCausalRandomForestRegressor
can be used to manage the balance between groups size (treatment and control) in each tree node. See here and hereNow
CausalTreeRegressor
and trees inCausalRandomForestRegressor
class can be plotted with the information about the treatment and control sample sizes that were used to build a tree. See from this line.Add tree visualization for CausalTreeRegressor #357
Example from jupyter notebook:
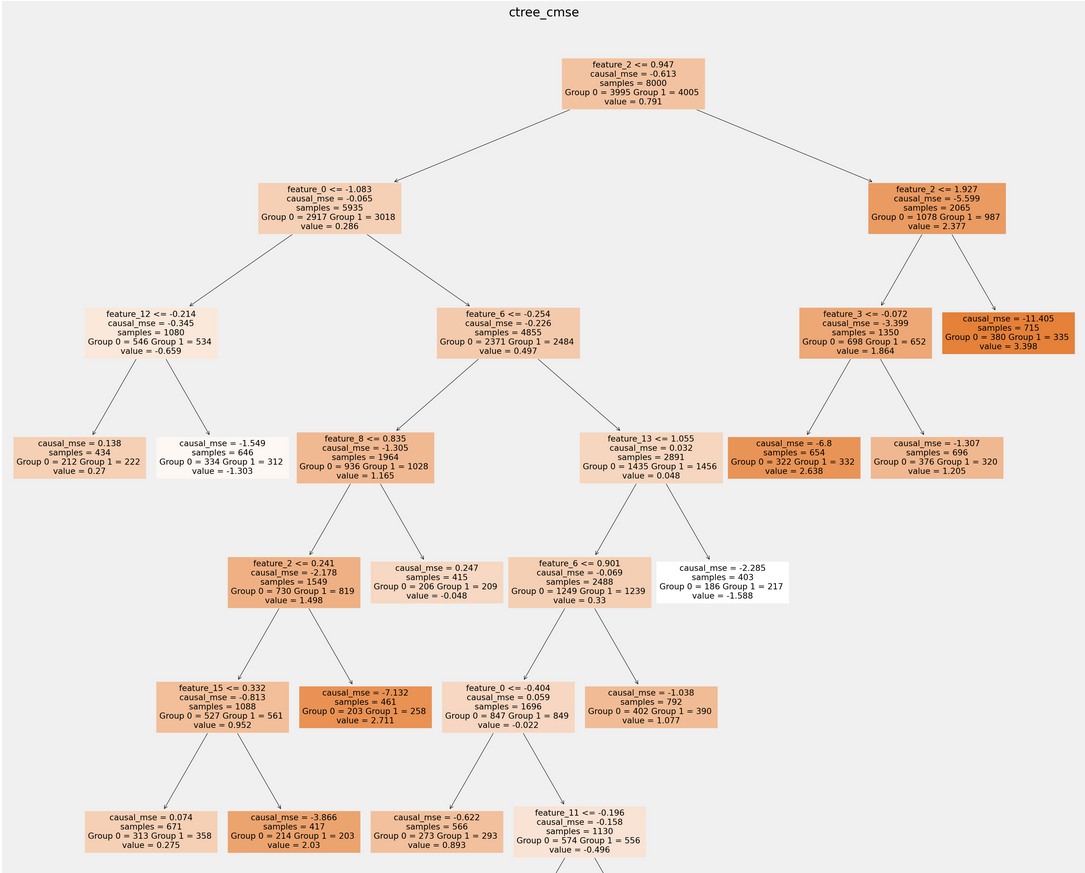
.pxd
files were added to move most third-party cython imports and auxulliary declarations.Although It's not quite fair to compare synthetic data results of this branch with the master, but causal trees were able to beat the previous successor
DRLearner
without much hyperparameters fine-tuning.Screenshots from
causal_trees_with_synthetic_data.ipynb
:Before:
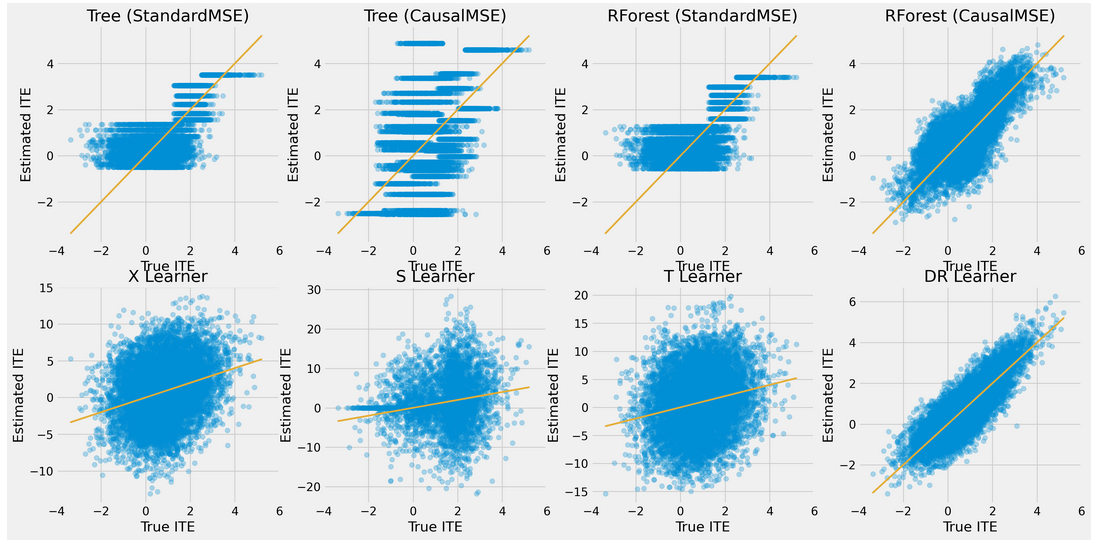
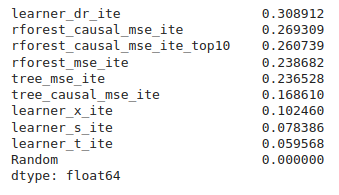
After the changes:
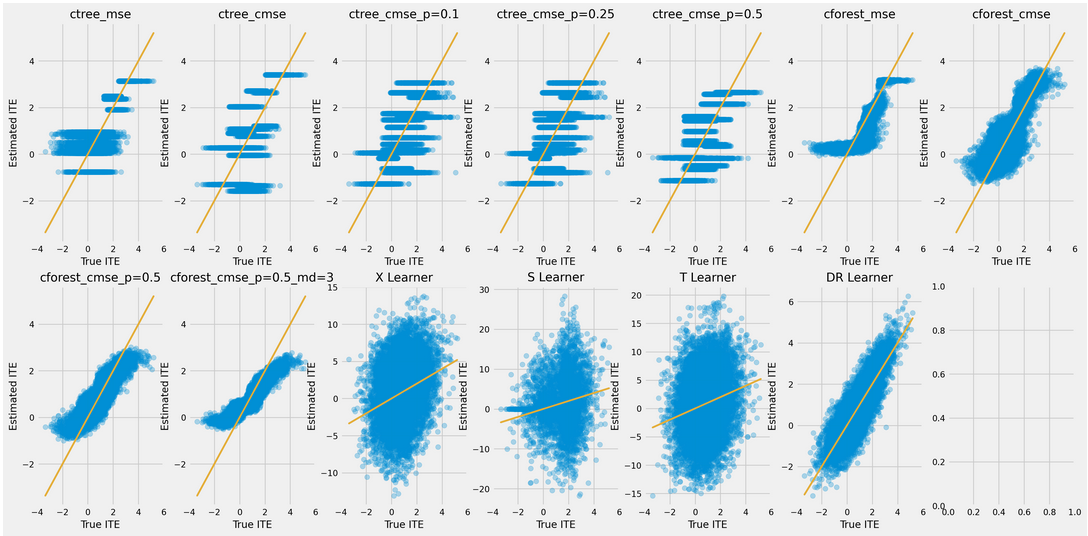
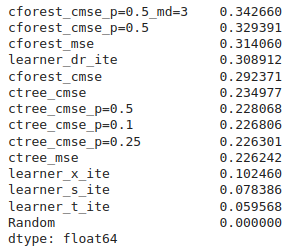
Types of changes
Checklist
Put an
x
in the boxes that apply. You can also fill these out after creating the PR. If you're unsure about any of them, don't hesitate to ask. We're here to help! This is simply a reminder of what we are going to look for before merging your code.Further comments
If this is a relatively large or complex change, kick off the discussion by explaining why you chose the solution you did and what alternatives you considered, etc. This PR template is adopted from appium.