-
Notifications
You must be signed in to change notification settings - Fork 11
New issue
Have a question about this project? Sign up for a free GitHub account to open an issue and contact its maintainers and the community.
By clicking “Sign up for GitHub”, you agree to our terms of service and privacy statement. We’ll occasionally send you account related emails.
Already on GitHub? Sign in to your account
Adiciona DDPG #45
Draft
Berbardo
wants to merge
2
commits into
main
Choose a base branch
from
ddpg
base: main
Could not load branches
Branch not found: {{ refName }}
Loading
Could not load tags
Nothing to show
Loading
Are you sure you want to change the base?
Some commits from the old base branch may be removed from the timeline,
and old review comments may become outdated.
Draft
Adiciona DDPG #45
Changes from 1 commit
Commits
Show all changes
2 commits
Select commit
Hold shift + click to select a range
File filter
Filter by extension
Conversations
Failed to load comments.
Loading
Jump to
Jump to file
Failed to load files.
Loading
Diff view
Diff view
There are no files selected for viewing
This file contains bidirectional Unicode text that may be interpreted or compiled differently than what appears below. To review, open the file in an editor that reveals hidden Unicode characters.
Learn more about bidirectional Unicode characters
Original file line number | Diff line number | Diff line change |
---|---|---|
@@ -0,0 +1 @@ | ||
{"nbformat":4,"nbformat_minor":0,"metadata":{"colab":{"name":"DDPG.ipynb","provenance":[],"collapsed_sections":[]},"kernelspec":{"name":"python3","display_name":"Python 3"},"accelerator":"GPU"},"cells":[{"cell_type":"markdown","metadata":{"id":"hjGWyD9Hyfg-"},"source":["# DDPG - Deep Deterministic Policy Gradient\n","\n","Deep Deterministic Policy Gradient (DDPG) é um algoritmo off-policy que aprende simultaneamente uma função Q-valor e uma política. Com a equação de Bellman, aprende a função Q e, com esta, aprende a política.\n","\n","A ideia de um algoritmo com gradiente da política surgiu primeiro com [Silver (sempre ele)](http://proceedings.mlr.press/v32/silver14.pdf), mas o algoritmo DDPG em si foi estabelecido na publicação [por Lilicrap](https://arxiv.org/abs/1509.02971)."]},{"cell_type":"markdown","metadata":{"id":"EXwpMV1OAnb_"},"source":["## O algoritmo de DDPG\n","\n","Este algoritmo é um Actor-Critic, mas, diferentemente dos que vimos antes, é off policy. Diferente do que estamos acostumados, este algoritmo só pode ser usado em espaços de ação contínuos. Podemos pensar então no DDPG como uma \"DQN para espaço de ação contínuo\".\n","\n","$$a^*(s) = arg max_a Q^*(s,a)$$\n","\n","A equação acima, da ação ótima para um dado estado, é familiar, mas se torna problemática para ações não discretas. É fácil contabilizar o máximo de uma série finita de ações, mas ações contínuas tornam essa operação problemática.\n","\n","Como sabemos que o espaço de ações é contínuo, podemos definir a política (ou função ator) $\\mu_\\theta(s) $, que vai nos ajudar a computar o max. Lembrando que, nesse algoritmo, a política é determinística.\n","\n","Dessa forma, temos então $max_a Q(s,a) \\approx Q(s, \\mu(s))$, facilitando a computação do max.\n","\n","### Q-Learning\n","\n","$$Q^*(s,a) = E_{s \\sim P} \\bigg[r(s,a) + \\gamma max_{a'} Q^*(s',a') \\bigg] $$\n","\n","A parte de Q learning, cuja equação para encontrar o valor ótimo de Q está acima, consiste em minimizar o erro quadrático médio de Bellman (MSBE), próximo do que já se era feito com a DQN.\n","\n","O [post da OpenAI](https://spinningup.openai.com/en/latest/algorithms/ddpg.html) oferece duas ferramentas para facilitar o treinamento da função Q, o uso de Replay Buffers e o uso de Target Networks. Uma vantagem de DDPG ser off-policy é permitir o uso de Replay Buffers.\n","\n","\n","### Policy Gradient\n","\n","O objetivo é achar a política determinística $\\mu_{\\theta}(s) $ que fornece a ação que maximiza $Q(s,a)$. Diferenciando a função Q em a, é possível então realizar gradiente ascendente para maximizar a política:\n","\n","$$\\nabla_\\theta J = \\mathbb{E}_{s_t} \\bigg[ \\nabla_a Q(s,a|\\theta^Q) |_{s = s_t, a = \\mu(s_t)} \\nabla_\\theta \\mu_\\theta(s|\\theta^\\mu)|_{s = s_t} \\bigg] $$\n","\n","\n","## Exploração\n","\n","Um problema para casos de ação contínua é a exploração. Por outro lado, por se tratar de um algoritmo off-policy, podemos tratar o problema da exploração independente do algoritmo de aprendizado. Dessa forma, podemos criar uma política de exploração $\\mu'$ adicionando ruído na nossa política/função ator:\n","\n","$$\\mu'(s) = \\mu_\\theta(s) + \\mathcal{N} $$\n","\n","No paper original, o ruído foi gerado por um processo [Ornstein-Uhlenbeck](https://en.wikipedia.org/wiki/Ornstein%E2%80%93Uhlenbeck_process). Porém, a openAI recomenda o uso de um ruído gaussiano não correlacionado e de média zero, por ser mais simples e funcionar tão bem quanto.\n","\n","\n","## Pseudocódigo\n","\n","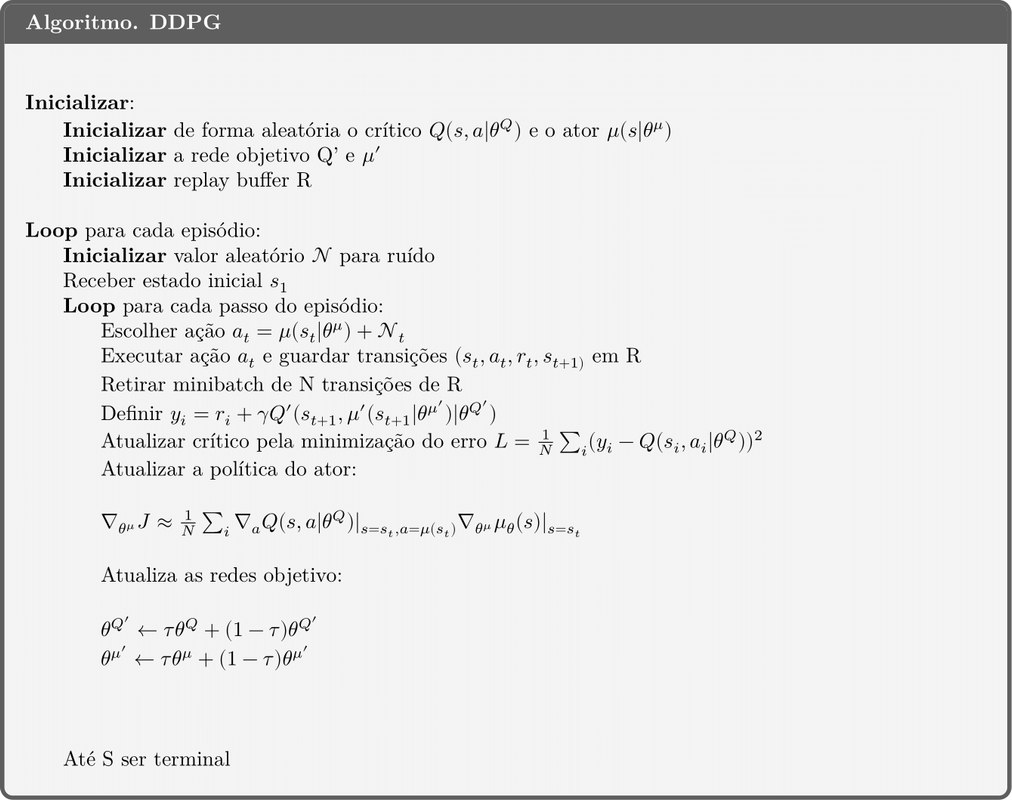\n","\n","\n","## Referências\n","https://spinningup.openai.com/en/latest/algorithms/ddpg.html\n","\n","http://proceedings.mlr.press/v32/silver14.pdf"]},{"cell_type":"markdown","metadata":{"id":"btLijj8y7gLH"},"source":["# Código\n"]},{"cell_type":"markdown","metadata":{"id":"YkTEyq25ms2B"},"source":["## Ator"]},{"cell_type":"code","metadata":{"id":"JOdt9KwNmcFR","executionInfo":{"status":"ok","timestamp":1606592473854,"user_tz":180,"elapsed":924,"user":{"displayName":"Bernardo Coutinho","photoUrl":"https://lh3.googleusercontent.com/a-/AOh14Gjbcuim7oGIm-uXpKRCJxDYg0Nhguq2a4_xKQcpjw=s64","userId":"08343358744938767290"}}},"source":["import torch\n","import torch.nn as nn\n","import torch.nn.functional as F\n","\n","class Actor(nn.Module):\n"," \"\"\"Rede do Ator.\"\"\"\n"," def __init__(self, observation_shape, action_shape, action_high, action_low):\n"," \"\"\"Inicializa a rede.\n"," \n"," Parâmetros\n"," ----------\n"," observation_shape: int\n"," Formato do estado do ambiente.\n"," \n"," action_shape: int\n"," Número de ações do ambiente.\n"," \"\"\"\n"," super(Actor, self).__init__()\n"," self.device = torch.device(\"cuda\" if torch.cuda.is_available() else \"cpu\")\n"," self.action_high = torch.from_numpy(action_high).to(self.device)\n"," self.action_low = torch.from_numpy(action_low).to(self.device)\n","\n"," self.linear1 = nn.Linear(observation_shape, 512)\n"," self.linear2 = nn.Linear(512, 256)\n"," self.linear3 = nn.Linear(256, action_shape)\n","\n"," def forward(self, state):\n"," \"\"\"\n"," Calcula a probabilidade de ação para o estado atual.\n"," \n"," Parâmetros\n"," ----------\n"," state: np.array\n"," Estado atual.\n"," \n"," Retorna\n"," -------\n"," action: torch.Tensor\n"," Ações.\n"," \"\"\"\n"," x = F.relu(self.linear1(state))\n"," x = F.relu(self.linear2(x))\n"," x = torch.tanh(self.linear3(x))\n"," \n"," action = x * (self.action_high - self.action_low) / 2.0 +\\\n"," (self.action_high + self.action_low) / 2.0\n","\n"," return action"],"execution_count":1,"outputs":[]},{"cell_type":"markdown","metadata":{"id":"TDbUOyZDmu6o"},"source":["## Crítico"]},{"cell_type":"code","metadata":{"id":"L2Ne0at-msW4","executionInfo":{"status":"ok","timestamp":1606592473857,"user_tz":180,"elapsed":920,"user":{"displayName":"Bernardo Coutinho","photoUrl":"https://lh3.googleusercontent.com/a-/AOh14Gjbcuim7oGIm-uXpKRCJxDYg0Nhguq2a4_xKQcpjw=s64","userId":"08343358744938767290"}}},"source":["import torch.nn as nn\n","import torch.nn.functional as F\n","\n","class Critic(nn.Module):\n"," \"\"\"Rede do Crítico.\"\"\"\n"," def __init__(self, observation_shape, action_shape):\n"," \"\"\"Inicializa a rede.\n"," \n"," Parâmetros\n"," ----------\n"," observation_shape: int\n"," Formato do estado do ambiente.\n","\n"," action_shape: int\n"," Número de ações do ambiente.\n"," \"\"\"\n"," super(Critic, self).__init__()\n","\n"," self.linear1 = nn.Linear(observation_shape, 512)\n"," self.linear2 = nn.Linear(512 + action_shape, 512)\n"," self.linear3 = nn.Linear(512, 300)\n"," self.linear4 = nn.Linear(300, 1)\n","\n"," def forward(self, state, action):\n"," \"\"\"\n"," Calcula o valor do estado atual.\n"," \n"," Parâmetros\n"," ----------\n"," state: np.array\n"," Estado atual.\n","\n"," state: np.array\n"," Ação escolhida.\n"," \n"," Retorna\n"," -------\n"," q: float\n"," Valor da ação escolhida.\n"," \"\"\"\n"," x = F.relu(self.linear1(state))\n"," xa_cat = torch.cat([x, action], 1)\n"," xa = F.relu(self.linear2(xa_cat))\n"," xa = F.relu(self.linear3(xa))\n"," q = self.linear4(xa)\n","\n"," return q"],"execution_count":2,"outputs":[]},{"cell_type":"markdown","metadata":{"id":"1ZiSoaCSnX-3"},"source":["## Experience Replay"]},{"cell_type":"code","metadata":{"id":"oh5ztI22nXiX","executionInfo":{"status":"ok","timestamp":1606592473859,"user_tz":180,"elapsed":918,"user":{"displayName":"Bernardo Coutinho","photoUrl":"https://lh3.googleusercontent.com/a-/AOh14Gjbcuim7oGIm-uXpKRCJxDYg0Nhguq2a4_xKQcpjw=s64","userId":"08343358744938767290"}}},"source":["import numpy as np\n","\n","class ExperienceReplay:\n"," \"\"\"Experience Replay Buffer para A2C.\"\"\"\n"," def __init__(self, max_length, observation_space):\n"," \"\"\"Cria um Replay Buffer.\n","\n"," Parâmetros\n"," ----------\n"," max_length: int\n"," Tamanho máximo do Replay Buffer.\n"," observation_space: int\n"," Tamanho do espaço de observação.\n"," \"\"\"\n"," self.index, self.length, self.max_length = 0, 0, max_length\n","\n"," self.states = np.zeros((max_length, *observation_space), dtype=np.float32)\n"," self.actions = np.zeros((max_length), dtype=np.int32)\n"," self.rewards = np.zeros((max_length), dtype=np.float32)\n"," self.next_states = np.zeros((max_length, *observation_space), dtype=np.float32)\n"," self.dones = np.zeros((max_length), dtype=np.float32)\n","\n"," def update(self, state, action, reward, next_state, done):\n"," \"\"\"Adiciona uma experiência ao Replay Buffer.\n","\n"," Parâmetros\n"," ----------\n"," state: np.array\n"," Estado da transição.\n"," action: int\n"," Ação tomada.\n"," reward: float\n"," Recompensa recebida.\n"," state: np.array\n"," Estado seguinte.\n"," done: int\n"," Flag indicando se o episódio acabou.\n"," \"\"\"\n"," self.states[self.index] = state\n"," self.actions[self.index] = action\n"," self.rewards[self.index] = reward\n"," self.next_states[self.index] = next_state\n"," self.dones[self.index] = done\n","\n"," self.index = (self.index + 1) % self.max_length\n"," if self.length < self.max_length:\n"," self.length += 1\n","\n"," def sample(self, batch_size):\n"," \"\"\"Retorna um batch de experiências.\n"," \n"," Parâmetros\n"," ----------\n"," batch_size: int\n"," Tamanho do batch de experiências.\n","\n"," Retorna\n"," -------\n"," states: np.array\n"," Batch de estados.\n"," actions: np.array\n"," Batch de ações.\n"," rewards: np.array\n"," Batch de recompensas.\n"," next_states: np.array\n"," Batch de estados seguintes.\n"," dones: np.array\n"," Batch de flags indicando se o episódio acabou.\n"," \"\"\"\n"," # Escolhe índices aleatoriamente do Replay Buffer\n"," idxs = np.random.randint(0, self.length, size=batch_size)\n","\n"," return (self.states[idxs], self.actions[idxs], self.rewards[idxs], self.next_states[idxs], self.dones[idxs])"],"execution_count":3,"outputs":[]},{"cell_type":"markdown","metadata":{"id":"-u-dalX7m-Co"},"source":["## Agente"]},{"cell_type":"code","metadata":{"id":"nNBne9yyr4vC","executionInfo":{"status":"ok","timestamp":1606592474496,"user_tz":180,"elapsed":1551,"user":{"displayName":"Bernardo Coutinho","photoUrl":"https://lh3.googleusercontent.com/a-/AOh14Gjbcuim7oGIm-uXpKRCJxDYg0Nhguq2a4_xKQcpjw=s64","userId":"08343358744938767290"}}},"source":["import numpy as np\n","import torch\n","from torch import optim\n","\n","class DDPG:\n"," def __init__(self, observation_space, action_space, pi_lr=0.001, q_lr=0.001, gamma=0.99, tau=0.005, action_noise=0.1):\n"," self.device = torch.device(\"cuda\" if torch.cuda.is_available() else \"cpu\")\n","\n"," self.gamma = gamma\n"," self.tau = tau\n","\n"," self.memory = ExperienceReplay(10000, observation_space.shape)\n","\n"," self.action_noise = action_noise\n"," self.action_shape = action_space.shape\n"," self.action_high = action_space.high\n"," self.action_low = action_space.low\n","\n"," self.actor = Actor(observation_space.shape[0], action_space.shape[0], self.action_high, self.action_low).to(self.device)\n"," self.target_actor = Actor(observation_space.shape[0], action_space.shape[0], self.action_high, self.action_low).to(self.device)\n","\n"," self.critic = Critic(observation_space.shape[0], action_space.shape[0]).to(self.device)\n"," self.target_critic = Critic(observation_space.shape[0], action_space.shape[0]).to(self.device)\n","\n"," for target_param, param in zip(self.target_critic.parameters(), self.critic.parameters()):\n"," target_param.data.copy_(param.data)\n","\n"," for target_param, param in zip(self.target_actor.parameters(), self.actor.parameters()):\n"," target_param.data.copy_(param.data)\n","\n"," self.actor_optimizer = optim.Adam(self.actor.parameters(), lr=pi_lr)\n"," self.critic_optimizer = optim.Adam(self.critic.parameters(), lr=q_lr)\n","\n"," def act(self, state):\n"," state = torch.FloatTensor(state).unsqueeze(0).to(self.device)\n"," \n"," with torch.no_grad():\n"," action = self.actor.forward(state)\n"," \n"," action = action.squeeze(0).cpu().numpy()\n"," action += self.action_noise * np.random.randn(*self.action_shape)\n","\n"," return np.clip(action, self.action_low, self.action_high)\n","\n"," def remember(self, state, action, reward, next_state, done):\n"," self.memory.update(state, action, reward, next_state, done)\n","\n"," def train(self, batch_size=32, epochs=1):\n"," if batch_size > self.memory.length:\n"," return\n"," \n"," for epoch in range(epochs):\n"," (states, actions, rewards, next_states, dones) = self.memory.sample(batch_size)\n","\n"," states = torch.FloatTensor(states).to(self.device)\n"," actions = torch.FloatTensor(actions).unsqueeze(-1).to(self.device)\n"," rewards = torch.FloatTensor(rewards).unsqueeze(-1).to(self.device)\n"," next_states = torch.FloatTensor(next_states).to(self.device)\n"," dones = torch.FloatTensor(dones).unsqueeze(-1).to(self.device)\n","\n"," self._train_critic(states, actions, rewards, next_states, dones)\n"," self._train_actor(states, actions, rewards, next_states, dones)\n"," self.update_target()\n","\n"," def _train_critic(self, states, actions, rewards, next_states, dones):\n"," q = self.critic.forward(states, actions)\n","\n"," with torch.no_grad():\n"," a2 = self.target_actor.forward(next_states)\n"," q2 = self.target_critic.forward(next_states, a2)\n"," \n"," target = rewards + self.gamma * q2\n"," critic_loss = F.mse_loss(q, target)\n","\n"," self.critic_optimizer.zero_grad()\n"," critic_loss.backward() \n"," self.critic_optimizer.step()\n","\n"," def _train_actor(self, states, actions, rewards, next_states, dones):\n"," policy_loss = -self.critic.forward(states, self.actor.forward(states)).mean()\n"," \n"," self.actor_optimizer.zero_grad()\n"," policy_loss.backward()\n"," self.actor_optimizer.step()\n","\n"," def update_target(self):\n"," with torch.no_grad():\n"," for target_param, param in zip(self.target_actor.parameters(), self.actor.parameters()):\n"," target_param.data.mul_(1 - self.tau)\n"," torch.add(target_param.data, param.data, alpha=self.tau, out=target_param.data)\n","\n"," for target_param, param in zip(self.target_critic.parameters(), self.critic.parameters()):\n"," target_param.data.mul_(1 - self.tau)\n"," torch.add(target_param.data, param.data, alpha=self.tau, out=target_param.data)"],"execution_count":4,"outputs":[]},{"cell_type":"markdown","metadata":{"id":"iu7U6Vmzoydm"},"source":["## Treinando"]},{"cell_type":"code","metadata":{"id":"zI1aZ7DypOfN","executionInfo":{"status":"ok","timestamp":1606592474498,"user_tz":180,"elapsed":1551,"user":{"displayName":"Bernardo Coutinho","photoUrl":"https://lh3.googleusercontent.com/a-/AOh14Gjbcuim7oGIm-uXpKRCJxDYg0Nhguq2a4_xKQcpjw=s64","userId":"08343358744938767290"}}},"source":["import math\n","from collections import deque\n","\n","def train(agent, env, total_timesteps):\n"," total_reward = 0\n"," episode_returns = deque(maxlen=20)\n"," avg_returns = []\n","\n"," state = env.reset()\n"," timestep = 0\n"," episode = 0\n","\n"," while timestep < total_timesteps:\n"," action = agent.act(state)\n"," next_state, reward, done, _ = env.step(action)\n"," agent.remember(state, action, reward, next_state, done)\n"," loss = agent.train()\n"," timestep += 1\n","\n"," total_reward += reward\n","\n"," if done:\n"," episode_returns.append(total_reward)\n"," episode += 1\n"," next_state = env.reset()\n","\n"," if episode_returns:\n"," avg_returns.append(np.mean(episode_returns))\n","\n"," total_reward *= 1 - done\n"," state = next_state\n","\n"," ratio = math.ceil(100 * timestep / total_timesteps)\n","\n"," avg_return = avg_returns[-1] if avg_returns else np.nan\n"," \n"," print(f\"\\r[{ratio:3d}%] timestep = {timestep}/{total_timesteps}, episode = {episode:3d}, avg_return = {avg_return:10.4f}\", end=\"\")\n","\n"," return avg_returns"],"execution_count":5,"outputs":[]},{"cell_type":"markdown","metadata":{"id":"cJIRJQ1NLVvV"},"source":["### Pendulum-v0\n","\n","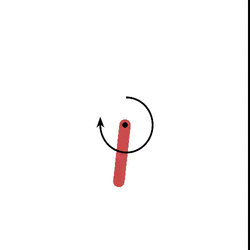"]},{"cell_type":"code","metadata":{"id":"1OGWuPmeox9X","executionInfo":{"status":"ok","timestamp":1606592474498,"user_tz":180,"elapsed":1548,"user":{"displayName":"Bernardo Coutinho","photoUrl":"https://lh3.googleusercontent.com/a-/AOh14Gjbcuim7oGIm-uXpKRCJxDYg0Nhguq2a4_xKQcpjw=s64","userId":"08343358744938767290"}}},"source":["import gym\n","\n","env = gym.make(\"Pendulum-v0\")"],"execution_count":6,"outputs":[]},{"cell_type":"code","metadata":{"id":"BMax8WJxo01m","executionInfo":{"status":"ok","timestamp":1606592478730,"user_tz":180,"elapsed":5777,"user":{"displayName":"Bernardo Coutinho","photoUrl":"https://lh3.googleusercontent.com/a-/AOh14Gjbcuim7oGIm-uXpKRCJxDYg0Nhguq2a4_xKQcpjw=s64","userId":"08343358744938767290"}}},"source":["agent = DDPG(env.observation_space, env.action_space)"],"execution_count":7,"outputs":[]},{"cell_type":"code","metadata":{"id":"IcWebqIBo2PG","colab":{"base_uri":"https://localhost:8080/"},"executionInfo":{"status":"ok","timestamp":1606592595686,"user_tz":180,"elapsed":122665,"user":{"displayName":"Bernardo Coutinho","photoUrl":"https://lh3.googleusercontent.com/a-/AOh14Gjbcuim7oGIm-uXpKRCJxDYg0Nhguq2a4_xKQcpjw=s64","userId":"08343358744938767290"}},"outputId":"f4bb8987-d3dd-416a-bccb-db5b32a09e35"},"source":["returns = train(agent, env, 15000)"],"execution_count":8,"outputs":[{"output_type":"stream","text":["[100%] timestep = 15000/15000, episode = 75, avg_return = -129.0745"],"name":"stdout"}]}]} | ||
Oops, something went wrong.
Add this suggestion to a batch that can be applied as a single commit.
This suggestion is invalid because no changes were made to the code.
Suggestions cannot be applied while the pull request is closed.
Suggestions cannot be applied while viewing a subset of changes.
Only one suggestion per line can be applied in a batch.
Add this suggestion to a batch that can be applied as a single commit.
Applying suggestions on deleted lines is not supported.
You must change the existing code in this line in order to create a valid suggestion.
Outdated suggestions cannot be applied.
This suggestion has been applied or marked resolved.
Suggestions cannot be applied from pending reviews.
Suggestions cannot be applied on multi-line comments.
Suggestions cannot be applied while the pull request is queued to merge.
Suggestion cannot be applied right now. Please check back later.
There was a problem hiding this comment.
Choose a reason for hiding this comment
The reason will be displayed to describe this comment to others. Learn more.
Seria legal colocar um gráfico do aumento da reward, assim como tem nos outros.