-
Notifications
You must be signed in to change notification settings - Fork 7
/
Copy pathtour-of-the-tidyverse.Rmd
488 lines (362 loc) · 13.1 KB
/
tour-of-the-tidyverse.Rmd
1
2
3
4
5
6
7
8
9
10
11
12
13
14
15
16
17
18
19
20
21
22
23
24
25
26
27
28
29
30
31
32
33
34
35
36
37
38
39
40
41
42
43
44
45
46
47
48
49
50
51
52
53
54
55
56
57
58
59
60
61
62
63
64
65
66
67
68
69
70
71
72
73
74
75
76
77
78
79
80
81
82
83
84
85
86
87
88
89
90
91
92
93
94
95
96
97
98
99
100
101
102
103
104
105
106
107
108
109
110
111
112
113
114
115
116
117
118
119
120
121
122
123
124
125
126
127
128
129
130
131
132
133
134
135
136
137
138
139
140
141
142
143
144
145
146
147
148
149
150
151
152
153
154
155
156
157
158
159
160
161
162
163
164
165
166
167
168
169
170
171
172
173
174
175
176
177
178
179
180
181
182
183
184
185
186
187
188
189
190
191
192
193
194
195
196
197
198
199
200
201
202
203
204
205
206
207
208
209
210
211
212
213
214
215
216
217
218
219
220
221
222
223
224
225
226
227
228
229
230
231
232
233
234
235
236
237
238
239
240
241
242
243
244
245
246
247
248
249
250
251
252
253
254
255
256
257
258
259
260
261
262
263
264
265
266
267
268
269
270
271
272
273
274
275
276
277
278
279
280
281
282
283
284
285
286
287
288
289
290
291
292
293
294
295
296
297
298
299
300
301
302
303
304
305
306
307
308
309
310
311
312
313
314
315
316
317
318
319
320
321
322
323
324
325
326
327
328
329
330
331
332
333
334
335
336
337
338
339
340
341
342
343
344
345
346
347
348
349
350
351
352
353
354
355
356
357
358
359
360
361
362
363
364
365
366
367
368
369
370
371
372
373
374
375
376
377
378
379
380
381
382
383
384
385
386
387
388
389
390
391
392
393
394
395
396
397
398
399
400
401
402
403
404
405
406
407
408
409
410
411
412
413
414
415
416
417
418
419
420
421
422
423
424
425
426
427
428
429
430
431
432
433
434
435
436
437
438
439
440
441
442
443
444
445
446
447
448
449
450
451
452
453
454
455
456
457
458
459
460
461
462
463
464
465
466
467
468
469
470
471
472
473
474
475
476
477
478
479
480
481
482
483
484
485
486
487
488
---
title: "An Antarctic Tour of the Tidyverse"
author: "Silvia P. Canelón, PhD (@spcanelon)"
date: "First created on Aug 31, 2020 (updated on Sept 22, 2020)"
institute: "University of Pennsylvania"
output:
html_notebook:
fig_width: 7.2
highlight: pygments
number_sections: no
theme: lumen
toc: yes
toc_float: yes
html_document:
toc: yes
df_print: paged
---
```{r}
knitr::opts_chunk$set(message = FALSE, warning = FALSE, collapse = TRUE)
```
# About {palmerpenguins}
- [{palmerpenguins} Documentation](https://allisonhorst.github.io/palmerpenguins/)
- [Allison Horst's GitHub repo for Palmer Penguins dataset](https://github.com/allisonhorst/palmerpenguins)
```{r, eval = FALSE}
# install.packages("remotes")
# remotes::install_github("allisonhorst/palmerpenguins")
```
# Loading packages
```{r}
# loading packages
library(tidyverse)
library(palmerpenguins)
# viewing data sets in package "palmerpenguins"
data(package = "palmerpenguins")
```
# readr
Let's get data into R!
```{r}
# option 1: load using URL ----
raw_adelie_url <- read_csv("https://portal.edirepository.org/nis/dataviewer?packageid=knb-lter-pal.219.3&entityid=002f3893385f710df69eeebe893144ff")
# option 2: load using filepath ----
raw_adelie_filepath <- read_csv("raw_adelie.csv")
```
Lucky for us, Allison Horst compiled data from all three species together for us in the `{palmerpenguins}` package!
- `penguins` contains a clean dataset, and
- `penguins_raw` contains raw data
```{r}
# saves package tibble into global environment
penguins <- palmerpenguins::penguins
head(penguins)
penguins_raw <- palmerpenguins::penguins_raw
head(penguins_raw)
```
# tibble
A `tibble` is much like the `data frame` in base R, but optimized for use in the Tidyverse. Let's take a look at the differences.
```{r tibble}
# try each of these commands in the console and see if you can spot the differences!
as_tibble(penguins)
as.data.frame(penguins)
```
# What differences do you see?
You might see a tibble prints:
- variable classes
- only 10 rows
- only as many columns as can fit on the screen
- NAs are highlighted in console so they're easy to spot (font highlighting and styling in `tibble`)
Not so much a concern in an R Markdown file, but noticeable in the console. Print method makes it easier to work with large datasets.
There are a couple of other main differences, namely in **subsetting** and **recycling**. Check them out in the [`vignette("tibble")](https://tibble.tidyverse.org/articles/tibble.html)
Try it out here!
```{r tibble-vignette}
vignette("tibble")
```
## Taking a closer look at `penguins`
Get a full view of the dataset:
```{r penguins-view}
View(penguins)
```
Or catch a `glimpse`:
```{r penguins-glimpse}
glimpse(penguins)
```
# ggplot2
Let's start by making a simple plot of our data!
`ggplot2` uses the "Grammar of Graphics" and layers graphical components together to create a plot.
## Let's see if body mass varies by penguin sex
```{r ggplot2}
penguins %>%
ggplot()
penguins %>%
ggplot(aes(x = sex, y = body_mass_g))
penguins %>%
ggplot(aes(x = sex, y = body_mass_g)) +
geom_point()
# A scatter plot doesn't really tell us much.
# Let's try a different geometry
penguins %>%
ggplot(aes(x = sex, y = body_mass_g)) +
geom_boxplot()
# That's more informative!
# Let's see if there are differences by penguin species
penguins %>%
ggplot(aes(x = sex, y = body_mass_g)) +
geom_boxplot(aes(fill = species))
# What do you notice?
```
## What observations can you make from the plot?
You might see:
- Gentoo penguins have higher body mass than Adelie and Chinstrap penguins
- Higher body mass among male Gentoo penguins compared to female penguins
- Pattern not as discernable when comparing Adelie and Chinstrap penguins
- No `NA`s among Chinstrap penguin data points! `sex` was available for each observation
I wonder what percentage of observations are `NA` for each species? Let's get the tidyverse to help us with this!
Next stop, `dplyr`!
# dplyr
```{r}
glimpse(penguins)
```
## select()
Selecting dataset columns with `select()`
```{r select}
penguins %>%
select(species, sex, body_mass_g)
```
## arrange()
Reordering the data set with `arrange()`
```{r arrange}
penguins %>%
select(species, sex, body_mass_g) %>%
arrange(desc(body_mass_g))
```
## group_by() and summarize()
Summarizing the data using `group_by()` and `summarize()`
We can use `group_by()` to group our data by **species** and **sex**, and `summarize()` to calculate the average **body_mass_g** for each grouping.
```{r group-by-summarize}
penguins %>%
select(species, sex, body_mass_g) %>%
group_by(species, sex) %>%
summarize(mean = mean(body_mass_g))
```
## count() and add_count()
If we're just interested in _counting_ the observations in each grouping, we can group and summarize with special functions `count()` and `add_count()`.
Counting can be done with `group_by()` and `summarize()`, but it's a little cumbersome.
It involves...
1. using `mutate()` to create an intermediate variable **n_species** that adds up all observations per **species**, and
2. an `ungroup()`-ing step
```{r}
penguins %>%
group_by(species) %>%
mutate(n_species = n()) %>%
ungroup() %>%
group_by(species, sex, n_species) %>%
summarize(n = n())
```
In contrast, `count()` and `add_count()` offer a simplified approach.
> Thank you to Alison Hill for [this suggestion](https://github.com/spcanelon/2020-rladies-chi-tidyverse/issues/2)!
```{r count}
penguins %>%
count(species, sex) %>%
add_count(species, wt = n,
name = "n_species")
```
## mutate()
We can add to our counting example by using `mutate()` to create a new variable **prop**, which represents the proportion of penguins of each **sex**, grouped by **species**
> Thank you to Alison Hill for [this suggestions](https://github.com/spcanelon/2020-rladies-chi-tidyverse/issues/2)!
```{r}
penguins %>%
count(species, sex) %>%
add_count(species, wt = n,
name = "n_species") %>%
mutate(prop = n/n_species*100)
```
## filter()
Finally, we can filter rows to only show us **Chinstrap** penguin summaries by adding `filter()` to our pipeline
```{r filter}
penguins %>%
count(species, sex) %>%
add_count(species, wt = n,
name = "n_species") %>%
mutate(prop = n/n_species*100) %>%
filter(species == "Chinstrap")
```
# forcats
Currently the `year` variable in `penguins` is continuous from 2007 to 2009.
There may be situations where this isn't what we want and we might want to turn it into a categorical variable instead.
The `factor()` function is perfect for this.
```{r}
penguins %>%
mutate(year_factor = factor(year, levels = unique(year)))
```
The result is a new factor `year_factor` with levels `2007`, `2008` and `2009`!
```{r}
penguins_new <-
penguins %>%
mutate(year_factor = factor(year, levels = unique(year)))
penguins_new
```
Double check the variable class and factor levels below:
```{r}
class(penguins_new$year_factor)
levels(penguins_new$year_factor)
```
# stringr
Let's play around with strings a little bit!
From what we've learned so far, take a guess at what this code chunk will do before running it.
```{r}
penguins %>%
select(species, island) %>%
mutate(ISLAND = str_to_upper(island))
```
How about this one? How is it different from the previous code chunk?
```{r}
penguins %>%
select(species, island) %>%
mutate(ISLAND = str_to_upper(island)) %>%
mutate(species_island = str_c(species, ISLAND, sep = "_"))
```
# tidyr
Both penguin datasets are already tidy!
We can pretend that it wasn't and that `body_mass_g` was recorded separately for `male`, `female`, and sex `NA` penguins. Like `untidy_penguins` below:
```{r}
untidy_penguins <-
penguins %>%
pivot_wider(names_from = sex,
values_from = body_mass_g)
untidy_penguins
```
Now let's make it tidy again with the help of the `pivot_longer()` function!
`pivot_wider()`is another very popular tidying function. Have you seen it before? Hint: see the code chunk above!
```{r}
untidy_penguins %>%
pivot_longer(cols = male:`NA`,
names_to = "sex",
values_to = "body_mass_g")
```
# purrr
Ok, we love our earlier boxplot showing us `body_mass_g` by `sex` and colored by `species`... but let's change up the colors to keep with our Antarctica theme!
I'm a big fan of the color palettes in the `nord` `r emo::ji("package")`
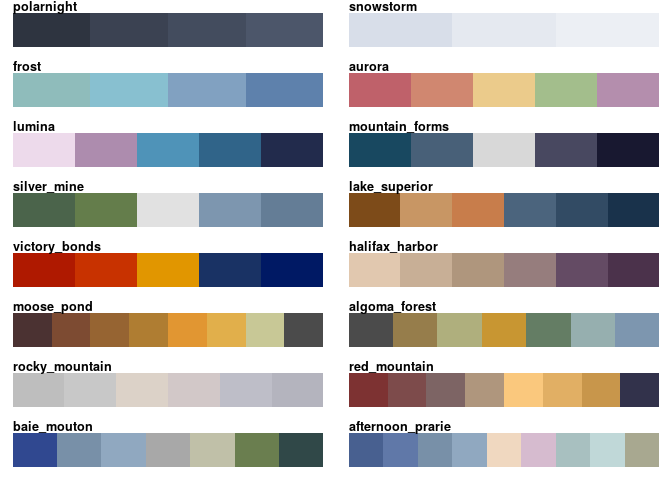
Let's turn this plot:
```{r}
penguins %>%
ggplot(aes(x = sex, y = body_mass_g)) +
geom_boxplot(aes(fill = species))
```
Into this one!
```{r}
penguins %>%
ggplot(aes(x = sex, y = body_mass_g)) +
geom_boxplot(aes(fill = species)) +
scale_fill_manual(values = nord::nord_palettes$frost)
```
Let's try out the `frost` palette.
```{r}
# we'll need to load the {nord} package
library(nord)
# you can choose colors using the color hex codes
nord::nord_palettes$frost
```
```{r}
# but you might prefer to use `scale_fill_manual()`
# or more specialized functions like `scale_fill_nord()`
# included in the {nord} package
penguins %>%
ggplot(aes(x = sex, y = body_mass_g)) +
geom_boxplot(aes(fill = species)) +
scale_fill_manual(values = nord::nord_palettes$frost)
#scale_fill_nord(palette = "frost")
```
Ok now for a handy package/function trio!
```{r}
# we'll have to load the {prismatic} package
library(prismatic)
prismatic::color(nord::nord_palettes$frost)
```
`purrr`'s `map()` function can help us iterate the `prismatic::color()` function over all palettes in a palette package like `nord`!
> Note: Not all colors will show well, like `polarnight` below. `prismatic::color()` relies on a package that kinda has limited functionality in this sense (`crayon`). It's doing its best :)
```{r, eval = FALSE}
nord::nord_palettes %>% map(prismatic::color)
```
# Extra material below!
## Recreating a {palmerpenguins} plot
Let's practice in real time!

```{r scatterplot-bill-length}
# scatterplot sequence ----
penguins %>%
ggplot() +
geom_point(aes(x = flipper_length_mm, y = bill_length_mm)) # add aesthetics
penguins %>%
ggplot() +
geom_point(aes(x = flipper_length_mm, y = bill_length_mm,
color = species)) # add color per species
penguins %>%
ggplot() +
geom_point(aes(x = flipper_length_mm, y = bill_length_mm,
color = species, shape = species)) # add shape per species
penguins %>%
ggplot() +
geom_point(aes(x = flipper_length_mm, y = bill_length_mm,
color = species, shape = species)) # add shape per species
penguins %>%
ggplot() +
geom_point(aes(x = flipper_length_mm, y = bill_length_mm,
color = species, shape = species)) +
geom_smooth(aes(x = flipper_length_mm, y = bill_length_mm,
color = species))
penguins %>%
ggplot(aes(x = flipper_length_mm, y = bill_length_mm)) +
geom_point(aes(color = species, shape = species)) +
geom_smooth(aes(color = species), se = FALSE, method = "lm")
```
```{r scatterplot-body-mass}
penguins %>%
ggplot() +
geom_point(aes(x = flipper_length_mm, y = body_mass_g,
color = species, shape = species))
```
```{r histogram}
penguins %>%
ggplot() +
geom_histogram(aes(x = flipper_length_mm))
penguins %>%
ggplot() +
geom_histogram(aes(x = flipper_length_mm, color = species))
penguins %>%
ggplot() +
geom_histogram(aes(x = flipper_length_mm, fill = species))
penguins %>%
ggplot() +
geom_histogram(aes(x = flipper_length_mm, fill = species,
position = "identity", alpha = 0.5))
```
# TidyTuesday
## Getting started
- [Intro Blog and history](https://themockup.blog/posts/2018-12-11-tidytuesday-a-weekly-social-data-project-in-r/)
- [Intro Tweet by Tom Mock (RStudio)](https://twitter.com/thomas_mock/status/1287774575833616384?s=20)
- [TidyTuesdays GitHub](https://github.com/rfordatascience/tidytuesday/blob/master/README.md)
- [TidyTuesday Podcast](https://twitter.com/tidypod)
- [TidyTuesday Sight-Unseen on YouTube](https://www.youtube.com/watch?v=ImpXawPNCfM)
- [{tidytuesdayR} package to help you easily download the data set](https://github.com/thebioengineer/tidytuesdayR)
## tidytuesdayR
```{r eval=FALSE}
# install.packages("tidytuesdayR")
# remotes::install_github("thebioengineer/tidytuesdayR")
library(tidytuesdayR)
# load the data
tt_data <- tt_load("2020-07-27") # error message
tt_data <- tt_load("2020-07-28")
tt_data <- tt_load(2020, week=31)
# take a peek
readme(tt_data)
print(tt_data)
```
## Level up with modeling
- [Julia Silge (RStudio) teaches Tidymodels with {palmerpenguins} on YouTube](https://www.youtube.com/watch?v=z57i2GVcdww)
## Examples
- Specific to {palmerpenguins}:
- Focus on fonts and formatting: https://twitter.com/ellamkaye/status/1289157139437621250?s=20
- R-Ladies Ames: https://twitter.com/RLadiesAmes/status/1294425359388114944?s=20
- General to #tidytuesday:
- R-Ladies Chicago TidyTuesday leader Ola!: https://twitter.com/AmazingSpeciali
- R-Ladies Chicago TidyTuesday setup: https://twitter.com/RLadiesChicago/status/1192243170412761088?s=20